Management
Safe and Sane: Understanding the FDA’s Risk-Based Approach to AI
Consider the example of a medical device manufacturer that needs to analyze customer complaints.
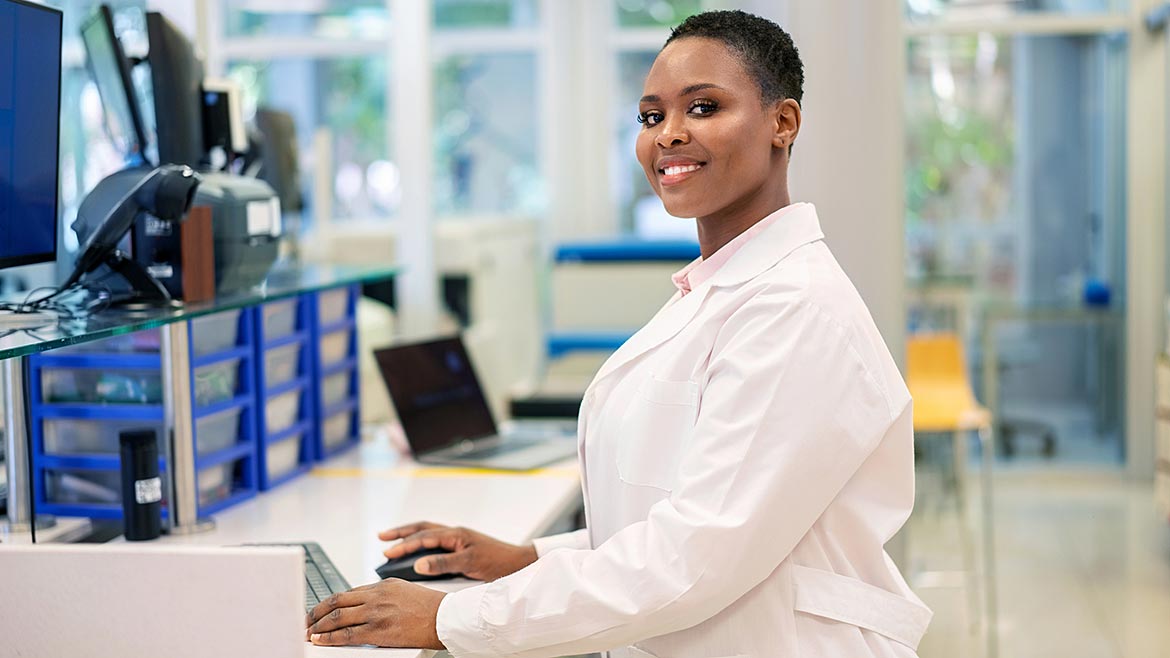
Image Source: alvarez / E+ / Getty Images Plus
The life sciences industry finds itself at a crossroads and the FDA just redrew the map for quality management in life sciences. AI isn’t just allowed—it’s expected.
While traditionally conservative in adopting new technologies, the sector now faces an explosion of AI capabilities in quality management systems. The FDA’s recent draft guidance, released in January 2025, provides a framework for evaluating and implementing AI technologies. After digesting the guidance and having worked closely with AI implementations in life sciences quality systems, it’s clear that successful implementation comes down to three fundamental questions.
Question 1: What Problem Do We Want AI to Solve?
The FDA’s guidance emphasizes that the first step in any AI implementation is critical thinking about intended use. Before considering any AI solution, quality managers must clearly define the specific problem they’re trying to solve, the current process and its pain points, and the desired role AI will play in the solution.
Consider this example: A medical device manufacturer needs to analyze customer complaints. They manufacture adhesive bandages and want the AI to identify patterns in complaints across multiple manufacturing sites. This application seems straightforward, but once the problem is identified, the risk level can change dramatically based on what role we give AI from there.
Question 2: What Role Will the AI Play in Decisions and Can It Handle that Role?
Quality professionals and manufacturers should ensure the AI model is used appropriately for the problem it is to solve. Understanding what data the model will consider is important. The role AI will play in decision making should also be documented. This helps regulators understand the limitations and the intended role of the AI model, and sets the foundation for risk assessment.
The FDA’s guidance indicates that the same AI capability can have vastly different risk classifications based on, among other things, its role in decision making.
Continuing with our bandage manufacturer example, two risk profiles emerge based on decision making:
- Lower Risk: AI analyzes complaints to suggest potential correlations between manufacturing changes and complaint patterns, which quality managers then investigate.
- Higher Risk: AI automatically triggers product recalls based on complaint pattern analysis without human verification.
Question 3: Do We Need a Human in the Loop?
The FDA’s guidance places significant emphasis on human oversight as a risk mitigation strategy. When implementing AI in your quality systems, you need to carefully consider the balance of decision-making authority. Will your AI system make autonomous decisions, or will it provide recommendations for human review? Understanding where and how human experts can intervene in the process is crucial for risk management.
Beyond direct oversight, the guidance emphasizes the importance of data diversification. Consider how many different data sources will inform your decisions and how human experts will be involved in interpreting this data. The FDA views AI recommendations as most valuable when they serve as one of multiple inputs in your decision-making process, rather than the sole determining factor.
In our bandage manufacturer example, a lower-risk implementation might look like this:
- The AI system identifies a pattern of skin irritation complaints
- The system notes correlations with a recent adhesive supplier change
- Quality managers review the analysis
- Quality teams query the system additional data (supplier records, test results)
- The team makes informed decisions about necessary actions
This approach uses AI as a tool to enhance human decision-making rather than replace it. The FDA guidance suggests that this type of implementation, with multiple data points and human oversight, generally carries lower risk and requires less extensive validation.
Implementing Your AI Strategy
Once you’ve answered these three key questions, your focus should shift to documentation and integration. Your documentation needs to include a clear description of intended use, risk assessment based on your specific use case, validation protocols appropriate to the risk level, and procedures for ongoing monitoring.
Integration requires careful consideration of how the AI solution fits into your existing quality systems. This means establishing training requirements for your quality team members, creating clear procedures for handling AI recommendations, and developing backup processes for times when the AI system might be unavailable.
Everyone in life sciences should be using AI but in a safe and sane way. The FDA’s new guidance gives us a framework to do exactly that. Start with low-risk implementations where AI serves as one voice among many in your decision-making process. Focus on use cases where a human remains firmly in the loop, and ensure your vendor provides clear data privacy controls and purpose-built solutions for life sciences. By approaching AI implementation thoughtfully and systematically, you can harness its benefits while maintaining the rigorous quality standards our industry demands and aligning with regulatory expectations.
Looking for a reprint of this article?
From high-res PDFs to custom plaques, order your copy today!