NDT | Computed Tomography
Real-time Quality Feedback Moves to the Active Production Line
Industrial CT-scanning systems and analysis software are providing faster insights into manufactured components.
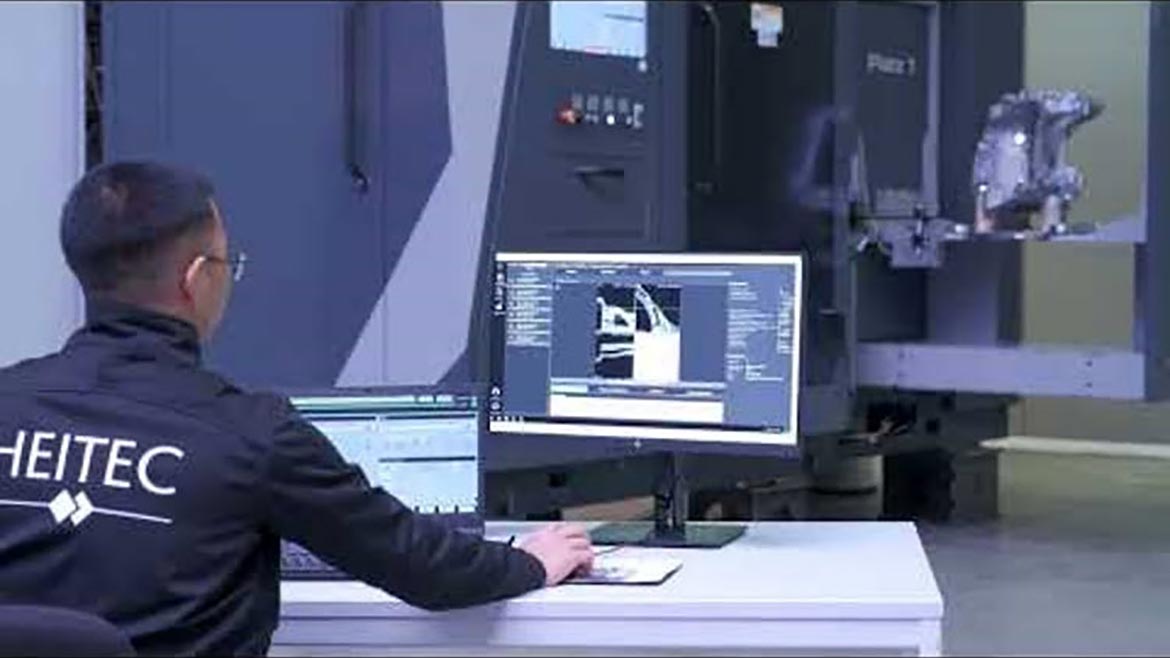
An engineer monitoring a robotic CT scan and analysis system as it inspects a manufactured component. Image courtesy of Heitec
Introduced by Henry Ford in 1913, the assembly line represented a major breakthrough in volume manufacturing. Yet, no matter what kind of product you are making, its final quality remains inexorably tied to the quality of those components being fed to it from production.
It follows that pre-assembly quality checks at key stages during active production are extremely important. As digital technology tools for such checks have become more accurate and efficient, increasing numbers of leading manufacturers—in automotive, aerospace, energy and electronics—are using computed tomography (CT) to inspect deep inside complex components to ensure they are fit to send to the assembly line.
CT scanning is expensive. But so is only finding out that costly components are defective after a product rolls off the assembly line. The savings achieved in time and resources when valuable parts are inspected for quality before they are assembled into a finished product can be significant.
Yet, until recently, the higher-resolution data capture of industrial-strength CT (which must sometimes penetrate far denser material than the human body tissues inspected by medical-grade scanning) has slowed the inspection process—taking up to an hour or more per item. Now, however, automation, analysis software, and even deep learning (a form of artificial intelligence, AI) are being brought together to slash inspection times to under a minute.
The secret’s in the scan—and the software
The solution to the time crunch? Employing lower-resolution industrial CT scans requiring significantly less time to capture an image—plus scanner-integrated software designed to process this “noisy” information and reconstruct the component’s volume data into a 3D computer model.
This data is then loaded into holistic analysis and visualization software that queries the digital model, employing sophisticated algorithms and even AI—to reliably identify, interpret, and report potential quality issues despite the noisy images—within a much shorter time window.

One of the leading types of this final analysis and visualization software options on the market has undergone over a quarter century of evolution and development, with process automation and, most recently, deep- and machine learning increasingly being integrated into its offerings. The huge quantities of part-quality data that can be captured by CT require this kind of a holistic approach, coupled with robust computational capabilities, to be most valuable. Rapid results delivery is critical for engineers looking for real-time insights into production-line quality as well as how manufacturing-parameter variations might be affecting outcomes.
Segmenting the data
Key to determining which data is significant in the scan of a component is the process of segmentation—the extraction of regions of interest (ROIs) from the 3D image data. Abrupt discontinuities in voxel grey-values typically indicate edges of discrete materials. By partitioning a scan into defined segments, the software can more rapidly focus on and catalogue the characteristics of the voxels in each segment. Segmentation thus enables the processing of only the important portions of a dataset—which takes a lot less time.


But how do you determine which segments are the important ones?
Especially since, in a lower-resolution CT scan obtained on a production line, the less-crisp image slices can be more challenging to segment. This is where deep- and machine-learning, forms of artificial intelligence (AI), comes into the picture: using trainable algorithms, AI can be harnessed to process and make segmentation decisions based on noisy CT data as accurately as on high-resolution data.
“Trainable” is the key word here. The algorithms compare what they see against an existing database of accurately segmented and labeled datasets specific to each manufacturer’s product geometries (which is where the “training” comes in). The algorithms “learn” to identify specific manufacturing flaws by accurately interpreting data regardless of their varying quality and resolution.
The number of training datasets (that have been segmented appropriately) needed to train a model well enough for proprietary requirements will vary from user to user. How complicated is the part? How many materials? How good is the data?
Customizing proprietary solutions with machine learning—a user example
One software customer, ELEMCA—an independent laboratory in France servicing manufacturing customers across multiple industries—recently saw marked time savings when putting this new functionality to work. They started by creating just 12 ideally segmented datasets (segmenting the four materials in the component being inspected). They fed these training sets into a trainer-software interface and ran the trainer for 15 hours to “learn” these skills and generate a model.


The model was then imported into the analysis software via a deep-segmentation module and subsequently run on any new dataset of that part. ELEMCA found that the model generated was sufficient to accurately segment the three materials in just 10 minutes per data set, rather than the hour it used to take manually. The company anticipates that the time savings of more than 80% that AI is delivering will help them improve customer satisfaction across a broader range of industries as they leverage these evolving capabilities.
The more data it is fed, the better deep learning performs; by comparing what it sees against real-world product data for which the solution is known, it learns to recognize patterns and features and call out deviations from the norm. In this way, it can provide highly accurate snapshots of what’s happening on a particular production line—supporting confident decision-making about accepting or rejecting a part. This informs production-variable changes, the effects of which can be captured, collated, and statistically examined.
How to optimize this kind of AI methodology is what forward-thinking manufacturers in various industries are in the process of discovering. Working closely under NDAs with robotics, scanning, and software providers, these companies are creating and curating their own internal data sets to train in-house deep learning systems.
Training a deep learning system to one’s proprietary data understandably takes manual time and effort. But it is paying off with scalability, accuracy, and resource savings on the production line. Actionable insights into what’s happening on one’s factory floor are contributing to the evolution of smart manufacturing, enabling companies across many industries to identify ways to improve quality while making their products more competitive.

Looking for a reprint of this article?
From high-res PDFs to custom plaques, order your copy today!