Artificial Intelligence (AI)
Case Studies of AI for Superhuman Quality Control in Electronics
The question is not whether manufacturers should adopt AI, but how quickly they can start reaping its benefits.

Image Source: SweetBunFactory / iStock / Getty Images Plus
Artificial intelligence (AI) is reshaping industries globally, and new innovations are unlocking benefits across the manufacturing sector. Quality control is one of the largest opportunities for the application of AI technologies. In a recent webinar titled “AI for Electronics Quality Control: Practical Applications & Case Studies,” I shared how machine learning and AI can revolutionize quality control in electronics manufacturing. I want to shed light on the profound impact these technologies have on improving processes, reducing costs, and enhancing product quality.
Key Technologies in the AI Toolbox
AI isn’t just one “thing,” it’s a collection of technologies – a toolbox filled with various tools, each suited for specific tasks. For the electronics manufacturing world, the most relevant tools are:
- Machine Learning (ML) and Deep Learning: These technologies enable manufacturers to analyze larger datasets and uncover insights that would be impossible to derive from statistical analysis alone. Machine learning can be used for tasks like defect classification, object detection, and anomaly detection. Machine learning can work with data sets that have 100 to 1,000 data points, while deep learning needs even larger datasets. In visual inspection tasks, both technologies can dramatically improve accuracy over conventional computer vision approaches.
- Large Language Models (LLMs): This is the technology behind ChatGPT and holds promise for enabling people to interact with their data or documents via a chat interface. However, the technology is relatively new and still generates words through a statistics engine, not from actually understanding the content.
- Generative AI (Gen AI): Generative AI is a relatively new technology that creates new media from prompts. There have been some attempts to use Gen AI to generate images of defects to then feed into ML or Deep Learning models, but I am skeptical on whether this would work outside of a proof of concept. We’ve found in our own research that even human-made defects can be problematic for training models if the defects aren’t truly representative of real failures – so I’m skeptical of generating defects for training.
- Math: Last but not least, basic statistics provides the foundation for all of the other pieces AI is built on. Statistics has widespread applications in quality control and is also a critical tool in the AI toolbox.
Quality Control Is The Best, First AI Application for Manufacturing
Manufacturing is complex and there are a lot of areas where AI might be able to improve core KPIs. In my view, quality control is the best AI use case to target first – for three reasons:
- Quality often has access to data. Quality data sets are large and well-structured, which creates an opportunity for AI to learn. However, even small data sets, like those collected from only 100 units, can still be enough for AI to provide benefits. Quality teams often have unique access to cross-functional and cross-supplier data sets to be fed into AI applications.
- Automate human visual inspection: AI can efficiently handle repetitive inspection tasks, which are often tedious and prone to human error. As I’ve pointed out, “Humans aren’t particularly great at inspection,” but it’s a place where AI can excel because once it’s been trained and tuned, it will perform consistently without getting tired. Leveraging AI for visual inspection can improve overall product quality while reducing the operational cost of the line.
- Quality is uniquely positioned to measure and quantify impact. Improving quality can significantly affect a business’s bottom line, so the return on investment (ROI) of investing in new technology is easier to demonstrate versus other places within the organization. Similarly, quality leaders can bridge the gap between operations and business metrics. They understand firsthand the costs associated with poor quality. General quality improvements have a significant impact on the bottom line—more so than engineering or operational efficiency improvements.
Practical Applications of AI to Improve Electronics Quality
One of the most common applications for AI in manufacturing is inspection. AI can drastically reduce inspection setup times and improve the accuracy of defect detection. Traditional computer vision (CV) systems require extensive setup and tedious rules programming. When changes are frequent, such as during NPI or when during periods of continuous improvement on the production line, CV systems are impractical because their rules need to be updated for each new version of the product. As a result, computer vision systems relying on CV are generally only used for “one issue, one camera” applications – where a specific failure mode is so financially painful that is justifies the entire cost of the camera and setup. Unfortunately, CV can only be used for identifying known issues. If you didn’t pre-program in the failure mode, the system won’t find it.
AI-based systems, on the other hand, can adapt to changes in the design or process in real-time. AI algorithms can be trained on datasets in just a few minutes, making AI easier to deploy and adapt. Additionally, they can detect both known and novel issues. For known issues, machine learning algorithms can outperform traditional methods by identifying defects with “superhuman” accuracy. The typical industry benchmark for human visual inspection is about 80% of defects caught – AI can catch up to 99%.
AI can also identify novel issues—those that haven’t been previously identified—catching defects that might otherwise slip through the cracks. The conventional approach for discovering novel issues is to have an engineer sit at the end of the line, looking at units and seeing if there’s anything not on the initial inspection procedure that needs to be added. But this relies on an engineer being in the right place at the right time – a bit of luck. And luck is not a strategy in quality.
An additional area where AI creates a significant business impact is on dark yield. Dark yield is made up of the defects you should have caught in the factory, but escaped – it’s dark because you cannot really measure how many units are escaping except for in field performance and complaints. A poorly mated connector or an assembly with less thermal paste than it should have might pass end-of-line functional tests, but may not hold up out in the field. Because AI can perform detection at higher levels than humans and can also find novel issues – it’s well-suited for revealing dark yield within the factory, which is a far better place to find defects than in the field.
Case Study: A Communications Leader Adopts AI
A $50 billion communications company that manufactures first-responder radios was experiencing quality escapes—defects that went undetected until the product was already in the field. The high stakes of this product, used by firefighters and other emergency responders, made quality control a top priority.
The company decided to implement AI-based inspection systems to catch defects that human inspectors were missing. By running a proof of concept with 1,000 units, they discovered that AI could detect critical defects—such as switched buttons and missing labels—that had gone unnoticed by human inspectors. The company was able to calculate its ROI for adding AI inspection easily. They were able to measure the reduction in inspection time and escape rate, how many fewer operators they needed, and what the break-even was. In this case, it was one month.

Demonstrate Proof of Value to Drive AI Adoption
Despite the clear benefits, adding AI to an existing operation comes with challenges. One of the biggest barriers is change management—convincing teams to embrace new technologies and integrate them into their workflows.
People don’t like change. It’s important to be thoughtful about how to introduce new technology to users such that they can see the value and impact on their personal work and KPIs, as well as the company’s KPIs. One way to convince both users and executive stakeholders is to run a Proof of Value pilot. Unlike a Proof of Concept, which tests the functionality of a technology, a Proof of Value tests the technology’s ability to move the needle on a critical KPI. By calculating the ROI of AI-based quality improvements and quantifying the cost of quality escapes, manufacturers can justify the investment and gain buy-in from key stakeholders.
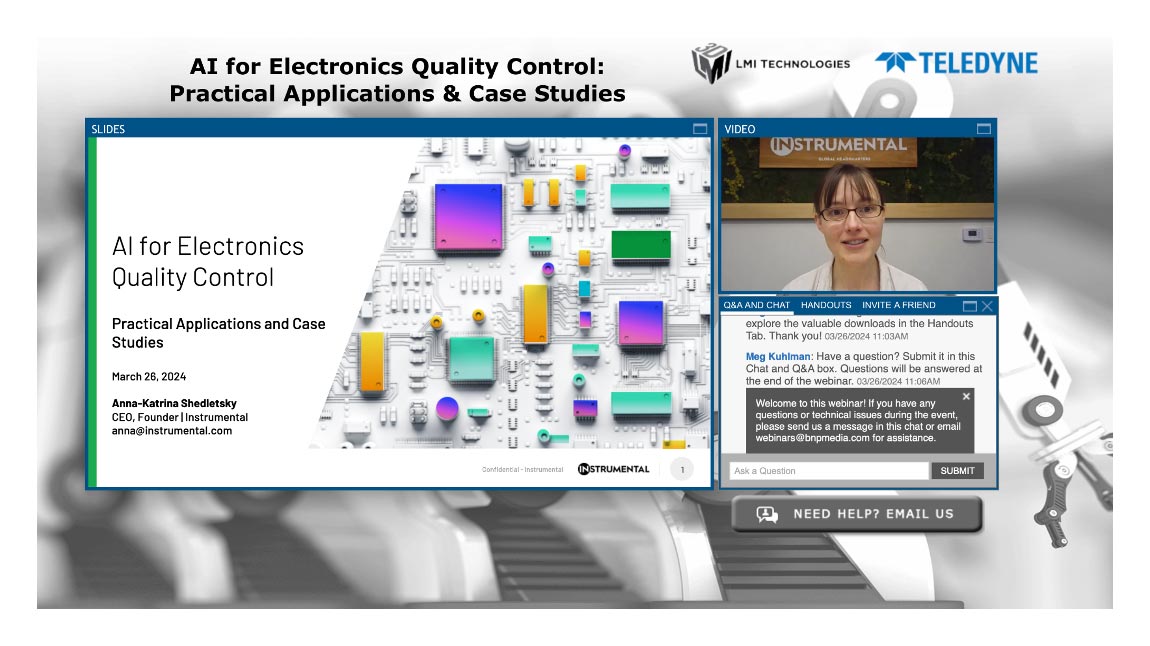
Conclusion: The Future of AI in Quality Control
AI is a powerful tool for improving quality control in manufacturing. With the ability to detect both known and unknown defects, automate repetitive tasks, and reduce costs, AI is poised to transform the way manufacturers ensure the quality of their products.
Quality leaders are best positioned to lead this transformation. AI is a great enhancement to existing conventional methods for quality control. Barriers to adoption can be overcome by building proof of value, where you can actually see this technology working.
The future of quality control is one where machines and humans work together to achieve unprecedented levels of precision and efficiency. The question is not whether manufacturers should adopt AI, but how quickly they can start reaping its benefits.
Looking for a reprint of this article?
From high-res PDFs to custom plaques, order your copy today!